What is agentic AI and how will it change contract management?
Discover how contract management leaders can leverage agentic AI to solve challenging problems and deliver substantial value.
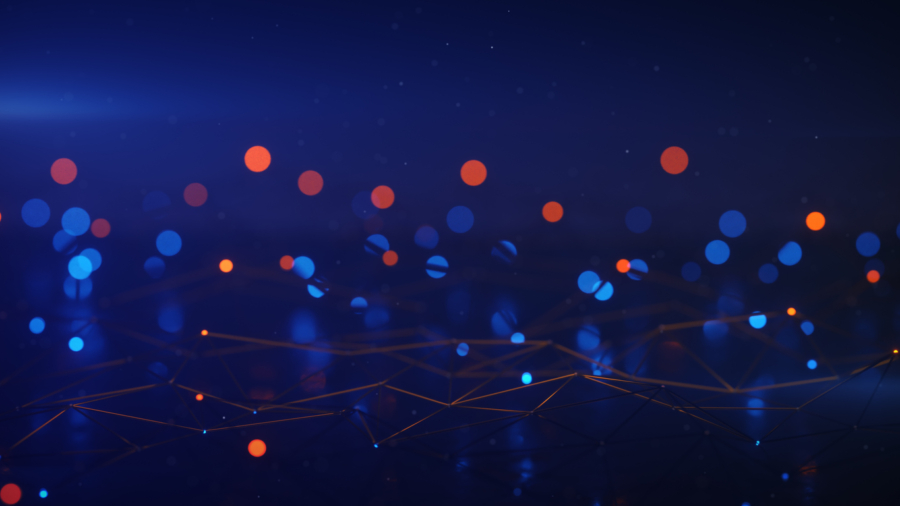
One of the buzziest buzzwords in technology today is agentic AI, but like most buzzwords, it’s only vaguely understood by most who encounter it.
At its core, agentic artificial intelligence (AI) refers to the use of AI – normally of the “generative AI” persuasion – to create and execute automation improvements using autonomous, self-directing AI code. Rather than simply responding to prompts with human-sounding answers, it gathers information, makes decisions, and executes activities.
For instance, an AI agent might:
- Seek to maintain a consistent revenue stream
- Monitor sales across regions
- Identify a trend in a particular location that is limiting revenue growth
- Check competitor prices to discover whether pricing is inhibiting sales
- Automatically adjust pricing where appropriate to boost revenue, then tweak it over time to achieve an optimal result
- Continue monitoring and adjusting to achieve its goal of revenue consistency
The key characteristics of an agentic AI solution are:
- Goal-oriented: The system isn’t simply monitoring and providing information, it is dedicated to delivering on a business outcome
- Decision-making: Often combining both AI and deterministic programming, an AI agent is designed to make decisions that actually change operations in pursuit of its goals
- Adaptability and continuous improvement: An agentic AI solution should not be statically-coded, but rather learn from the outcomes of the decisions it makes to continuously improve future activities
- Interoperability: Whether it’s simply monitoring across its own organizations’ systems, or also tapping into the collected intelligence available across the open Web, an AI agent should be able to use information and perspective from a variety of sources to inform its decisions
- Autonomy: Once enabled, agentic AI executes its pursuit of goals without constant manual intervention
The state of agentic AI in technology today
There aren’t many true agentic AI solutions out there yet, but plenty are coming close. Organizations have been using data mining for years to spot trends and tweak operations, usually with pre-programmed routines. While these don’t quite fit the agentic AI category, any AI used in ongoing, automated decision-making today should probably be worthy of the monicker.
Why? Because most organizations remain wary of letting AI take truly autonomous actions. For a generation of workers that is constantly frustrated by the terrible interactions they have with AI-driven customer service and embarrassed by the stunningly incorrect autocorrect features on their smart phones, the idea of giving up human control to a machine feels very uncomfortable.
Consider the classic example of how agentic AI ‘should’ operate. In this example, a traveler tells the AI that they want to take a trip somewhere – say, a weeklong journey to the Midwest to see three baseball games at different, but relatively close-by, stadiums. The idea is that the AI, knowing the user’s preferences, will research the best options, then book their flights, rental car, baseball tickets, hotels, dinner reservations, then provide an ideal itinerary back to the user.
While this does sound glorious, that’s a lot of power to cede to an algorithm – not least when it needs access to the user’s credit card to make the bookings! One little error (and AI is well-known for its hallucinations and made-up facts), and the traveler could find themselves with non-refundable tickets to the wrong sport, driving an ultra-luxury vehicle hundreds of miles between budget motels.
So, today’s agentic AI doesn’t tend to check all the characteristic boxes of the ideal – but that’s not to say it doesn’t exist.
Using AI to build agents that make decisions within pre-defined parameters should still be considered agentic AI, so long as at least three of the characteristics are met. In our first example of the revenue-optimization agent, for instance, we might allow the system to monitor revenue streams, to compare those to competitors, and to adjust prices – but with a human in the loop, approving changes, to ensure revenue growth isn’t being boosted at the cost of negative margins. This example still uses AI to improve the process but removed its autonomy to avoid unforeseen problems.
Of course, the challenge with lowering the standards of what counts as truly “agentic” AI raises the likelihood of fudging by unscrupulous actors. The concept of “agent washing” has become a much-debated topic in the business world: simply slapping the words agentic AI on a product does not make it so.
A chatbot that answers questions based on a fixed knowledge base is not truly agentic. Certainly, it uses AI to interpret the natural language questions it receives to find the answer and to respond to the user in a human-like manner. However, it is not truly goal-oriented (simply answering questions doesn’t really count as a pursuable goal); it is not autonomous; and it is dipping into only a single pool of knowledge to achieve its targets.
How agentic AI can enhance CLM software
Taking this all into account, contract management leaders looking to solve their most challenging problems with agentic AI need to both avoid agent-washed products but also be open to solutions that deliver value within the narrower confines of what is truly possible.
As professionals who greatly value accuracy and precision, those who process and approve contracts are necessarily leery of giving up control over the language that populates their documents. At the same time, they often find themselves with a mismatch between time spent on routine activities and value delivered. For example, an initial contract review may force legal professionals to spend hours reading innocuous language just to uncover a couple of knotty issues that need fixing. Or, they may find themselves dedicating an unseemly amount of time assessing the complexity of a given contract just to decide who needs to be on the approval chain.
These routine procedures are perfect opportunities for AI agents to step in and deliver substantial time savings. One Agiloft customer, for instance, built a GenAI action that evaluates uploaded contracts and automatically assigns them to the right approval sequence, accelerating time to completion and saving hours of grinding effort that could be better spent actually negotiating details. Others use built-in agents to autonomously build summaries to make complex agreements easier to understand for their non-Legal colleagues.
AI agents like these comply with three of the five core characteristics of agentic AI, including:
- Goal-oriented: Delivering something of value without guidance beyond the original prompt
- Decision-making: Interpreting the contents of a document to drive decisions, then making actual changes to process and communications
- Autonomous: Will work independently until told to stop, although they can be adjusted as needed
Looking ahead to the future
While the time may someday come where contracting professionals become content to hand over complete control of their contracts to AI, that time is not yet upon us. But by building AI agents that meet at least three characteristic criteria of agentic AI, they can accelerate their processes, reduce their manual work, and drive superior results overall.
Agiloft puts AI at the core of everything we create for all of our customers. Learn more about how we put AI on the inside™ in our blog post here.
Recent
Posts
Learn about the realities of AI today, its limitations and capabilities, and its use as a “force multiplier” for contracting.
If there is one message for tech buyers as we approach 2024, it is that AI is here – ready or not.
With the introduction of ConvoAI, Agiloft delivers the same benefits of simplified AI experiences to the world of contracts.